Algorithm Integrity Matters
Algorithm Integrity Matters
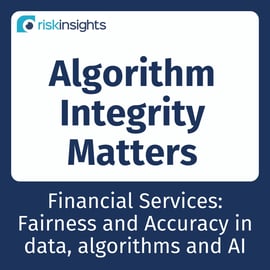
A podcast for FS leaders who want to enhance fairness and accuracy in their use of data, algorithms, and AI.
Each episode explores existing and emerging challenges and solutions related to algorithmic integrity, including discussions on navigating independent audits.
The goal of this podcast is to give leaders the knowledge they need to ensure their data practices benefit customers and other stakeholders, reducing the potential for harm and upholding industry standards.
Guest Interview Episodes
Episode 21:
Ryan Carrier, Executive Director of ForHumanity
Ryan Carrier is founder and executive director of ForHumanity, a non-profit focused on mitigating the risks associated with AI, autonomous, and algorithmic systems.
With 25 years of experience in financial services, Ryan discusses ForHumanity's mission to analyze and mitigate the downside risks of AI to benefit society.
The conversation includes insights on the foundation of ForHumanity, the role of independent AI audits, educational programs offered by the ForHumanity AI Education and Training Center, AI governance, and the development of audit certification schemes.
Ryan also highlights the importance of AI literacy, stakeholder management, and the future of AI governance and compliance.
The full transcript of this episode is available here.
Episode 22:
Patrick Sullivan, VP of Strategy and Innovation at A-LIGN
Patrick Sullivan is Vice President of Strategy and Innovation at A-LIGN and an expert in cybersecurity and AI compliance with over 25 years of experience.
Patrick shares his career journey, discusses his passion for educating executives and directors on effective governance, and explains the critical role of management systems like ISO 42001 in AI compliance.
We discuss the complexities of AI governance, risk assessment, and the importance of clear organizational context.
Patrick also highlights the challenges and benefits of AI assurance and offers insights into the changing landscape of AI standards and regulations.
The full transcript of this episode is available here.
Episode 24:
Shea Brown, Founder and CEO of BABL AI
Dr. Shea Brown is Founder and CEO of BABL AI
BABL specializes in auditing and certifying AI systems, consulting on responsible AI practices, and offering online education.
Shea shares his journey from astrophysics to AI auditing, the core services provided by BABL AI including compliance audits, technical testing, and risk assessments, and the importance of governance in AI.
He also addresses the challenges posed by generative AI, the need for continuous upskilling in AI literacy, and the role of organizations like the IAAA and For Humanity in building consensus and standards in AI auditing.
Finally, Shea provides insights on third-party risks, in-house AI developments, and key skills needed for effective AI governance.
The full transcript of this episode is available here.
List of (all) Episodes
Episode 0:
Introduction
A brief intro to the podcast.
If you have topic or guest suggestions, feel free to reach out via email: info@riskinsights.com.au
Episode 1:
How reliable is the algorithm / workflow audit that you have commissioned?
Spoken (by a human) version of this article.
One common issue with audits is undue reliance.
Can you rely on the audit report to tell you what you need to know?
Could you be relying on it too much?
Episode 2:
Choice vs obligation: motivation shapes the effectiveness of your audit
Spoken (by a human) version of this article.
The motivation(s) for commissioning an audit can determine how effective it will be.
Often, our approach differs depending on whether we are forced to, or choose to. Our engagement and satisfaction levels are generally higher when we choose (than when we are forced).
Episode 3:
Navigate Algorithm Audit Guidance: some aren't relevant to your context
Spoken (by a human) version of this article.
AI and algorithm audits help ensure ethical and accurate data processing, preventing harm and disadvantage.
However, the guidelines are not yet mature, and quite disparate.
This can make the audit process confusing, and quite daunting - how do you wade through it all to find the information that you need, in deciding how to commission your audit?
Fortunately, there is a solution - narrowing the guidelines down, based on relevance.
Not all existing guidelines are universally applicable.
This article will help you distinguish between audit guidance that applies to your situation and guidance that may not be relevant to your industry, deployment, or organizational needs.
Episode 4:
Structuring the Audit Objective: 10 Key Aspects of Algorithm Integrity
Spoken (by a human) version of this article.
In Episode 1, we explored the challenges of placing undue reliance on audits.
One potential solution that we outlined is a clear scope, particularly regarding the audit objective.
In this episode, we focus on algorithm integrity as the broad audit objective.
While it’s easy to assert that an algorithm has integrity, confirming this assertion is a bit more complex.
To help simplify this, this episode breaks it down into a set of key areas to consider.
Episode 5:
Equal vs Equitable: Algorithmic Fairness
Spoken (by a human) version of this article.
Fairness in algorithmic systems is a multi-faceted, and developing, topic. In episode 4, we explored ten key aspects to consider when scoping an algorithm integrity audit.
One aspect was fairness, with this in the description: "...The design ensures equitable treatment..."
This raises an important question. Shouldn't we aim for equal, rather than equitable treatment?
This episode aims to shed light on the distinctions between equal and equitable treatment in algorithmic systems, while acknowledging that our understanding of fairness is still developing and subject to ongoing debate.
Episode 6:
Balancing Security and Access for increased algorithmic integrity
Spoken (by a human) version of this article.
When we talk about security in algorithmic systems, it's easy to focus solely on keeping the bad guys out.
But there's another side to this coin that's just as important: making sure the right people can get in.
This article aims to explain how security and access work together for better algorithm integrity.
Episode 7:
Postcodes: Hidden Proxies for Protected Attributes
Spoken (by a human) version of this article.
In a previous article, we discussed algorithmic fairness, and how seemingly neutral data points can become proxies for protected attributes.
In this article, we'll explore a concrete example of a proxy used in insurance and banking algorithms: postcodes.
We've used Australian terminology and data. But the concept will apply to most countries.
Using Australian Bureau of Statistics (ABS) Census data, it aims to demonstrate how postcodes can serve as hidden proxies for gender, disability status and citizenship.
Episode 8:
A Balanced Focus on New and Established Algorithms
Spoken (by a human) version of this article.
Even in discussions among AI governance professionals, there seems to be a silent “gen” before AI.
With rapid progress - or rather prominence – of generative AI capabilities, these have taken centre stage.
Amidst this excitement, we mustn't lose sight of the established algorithms and data-enabled workflows driving core business decisions. These range from simple rules-based systems to complex machine learning models, each playing a role in our operations.
In this episode, we'll examine why we need to keep an eye on established algorithmic systems, and how.
Episode 9:
Algorithmic Integrity: Don't wait for legislation
Spoken (by a human) version of this article.
Legislation isn't the silver bullet for algorithmic integrity.
Are they useful? Sure. They help provide clarity and can reduce ambiguity. And once a law is passed, we must comply.
However, existing legislation may already apply, new algorithm-focused laws can be too narrow or quickly outdated, etc.
In short, they are helpful, but we need to know what we're getting - what they cover, don't cover, etc.
Episode 10:
Fairness reviews: identifying essential attributes
Spoken (by a human) version of this article.
When we're checking for fairness in our algorithmic systems (incl. processes, models, rules), we often ask:
What are the personal characteristics or attributes that, if used, could lead to discrimination?
This article provides a basic framework for identifying and categorising these attributes.
Episode 11:
Deprovisioning User Access to Maintain Algorithm Integrity
Spoken (by a human) version of this article.
The integrity of algorithmic systems goes beyond accuracy and fairness.
In Episode 4, we outlined 10 key aspects of algorithm integrity.
Number 5 in that list (not in order of importance) is Security: the algorithmic system needs to be protected from unauthorised access, manipulation and exploitation.
In this episode, we explore one important sub-component of this: deprovisioning user access.
Link from article: U.S. National Coordinator for Critical Infrastructure Security and Resilience (CISA) advisory.
Episode 12:
Risk-Focused Principles for Change Control in Algorithmic Systems
Spoken (by a human) version of this article.
With algorithmic systems, an change can trigger a cascade of unintended consequences, potentially compromising fairness, accountability, and public trust.
So, managing changes is important. But if you use the wrong framework, your change control process may tick the boxes, but be both ineffective and inefficient.
This article outlines a potential solution: a risk focused, principles-based approach to change control for algorithmic systems.
Resource mentioned in the article: ISA 315 guideline for general IT controls.
Episode 13:
Bridging the purpose-risk gap: Customer-first algorithmic risk assessments
Spoken (by a human) version of this article.
Banks and insurers sometimes lose sight of their customer-centric purpose when assessing AI/algorithm risks, focusing instead on regular business risks and regulatory concerns.
Regulators are noticing this disconnect.
This article aims to outline why the disconnect happens and how we can fix it.
Report mentioned in the article: ASIC, REP 798 Beware the gap: Governance arrangements in the face of AI innovation.
Episode 14:
External data - use with care
Spoken (by a human) version of this article.
Banks and insurers are increasingly using external data; using them beyond their intended purpose can be risky (e.g. discriminatory).
Emerging regulations and regulatory guidance emphasise the need for active oversight by boards, senior management to ensure responsible use of external data.
Keeping the customer top of mind, asking the right questions, and focusing on the intended purpose of the data, can help reduce the risk.
Law and guideline mentioned in the article:
- Colorado's External Consumer Data and Information Sources (ECDIS) law
- New York's proposed circular letter.
Episode 15:
Algorithm Integrity Documentation - Getting Started
Spoken (by a human) version of this article.
Documentation makes it easier to consistently maintain algorithm integrity.
This is well known.
But there are lots of types of documents to prepare, and often the first hurdle is just thinking about where to start.
So this simple guide is meant to help do exactly that – get going.
Episode 16:
Algorithmic System Accuracy Reviews – Choosing the Right Approach
Spoken (by a human) version of this article.
Outcome-focused accuracy reviews directly verify results, offering more robust assurance than process-focused methods.
This approach can catch translation errors, unintended consequences, and edge cases that process reviews might miss.
While more time-consuming and complex, outcome-focused reviews provide deeper insights into system reliability and accuracy.
This article explains why verifying outcomes is preferred over tracing through processes, and how it works.
Episode 17:
Algorithm Integrity: Audit vs Review
Spoken (by a human) version of this article.
The terminology – “audit” vs “review” - is important, but clarity about deliverables is more important when commissioning algorithm integrity assessments.
Audits are formal, with an opinion or conclusion that can often be shared externally. Reviews come in various forms and typically produce recommendations, for internal use.
Regardless of the terminology you use, when commissioning an assessment, clearly define and document the expected deliverable, including the report content and intended distribution, to ensure expectations are met.
Episode 18:
Algorithm Integrity: Training and Awareness
Spoken (by a human) version of this article.
Ongoing education helps everyone understand their role in responsibly developing and using algorithmic systems.
Regulators and standard-setting bodies emphasise the need for AI literacy across all organisational levels.
Links
- ForHumanity - join the growing community here.
- ForHumanity - free courses here.
- IAIS: The International Association of Insurance Supervisors - guidance paper.
- DNB: De Nederlandsche Bank - 6 general principles for the use of AI in the financial sector.
- ASIC: The Australian Securities & Investments Commission - report.
- NIST: The National Institute of Standards and Technology - AI Risk Management Framework.
- EU AI Act: The EU Artificial Intelligence Act - specific expectation about “AI literacy”.
Episode 19:
Algorithmic System Reviews: Substantive vs. Controls Testing
Spoken (by a human) version of this article.
- Knowing the basics of substantive testing vs. controls testing can help you determine if the review will meet your needs.
- Substantive testing directly identifies errors or unfairness, while controls testing evaluates governance effectiveness. The results/conclusions are different.
- Understanding these differences can also help you anticipate the extent of your team's involvement during the review process.
Episode 20:
Algorithm Reviews: Public vs Private Reports
Spoken (by a human) version of this article.
- Public AI audit reports aren't universally required; they mainly apply to high-risk applications and/or specific jurisdictions.
- The push for transparency primarily concerns independent audits, not internal reviews.
- Prepare by implementing ethical AI practices and conducting regular reviews.
Note: High-risk AI systems in banking and insurance are subject to specific requirements
Episode 23:
AI Risk Training: Role-based tailoring
Spoken (by a human) version of this article.
- AI literacy is growing in importance (e.g., EU AI Act, IAIS).
- AI literacy needs vary across roles..
- Even "AI professionals" need AI Risk training.
Episode 25:
Algorithm Integrity: Third party assurance
Spoken (by a human) version of this article.
- Third-party assurance for algorithm integrity varies based on the nature of the relationship and specific needs, with several options.
- Key factors to consider include the importance and risk level of the service/product, regulatory expectations, complexity, transparency, and frequency of updates.
- Standardised assurance frameworks for algorithm integrity are still emerging; adopt a risk-based approach, and consider sector-specific standards like CPS230(Australia).
Episode 26:
Algorithmic System Integrity: Testing
Spoken (by a human) version of this article.
- Testing is a core basic step for algorithmic integrity.
- Testing involves various stages, from developer self-checks to UAT. Where these happen will depend on whether the system is built in-house or bought.
- Testing needs to cover several integrity aspects, including accuracy, fairness, security, privacy, and performance.
- Continuous testing is needed for AI systems, differing from traditional testing due to the way these newer systems change (without code changes).
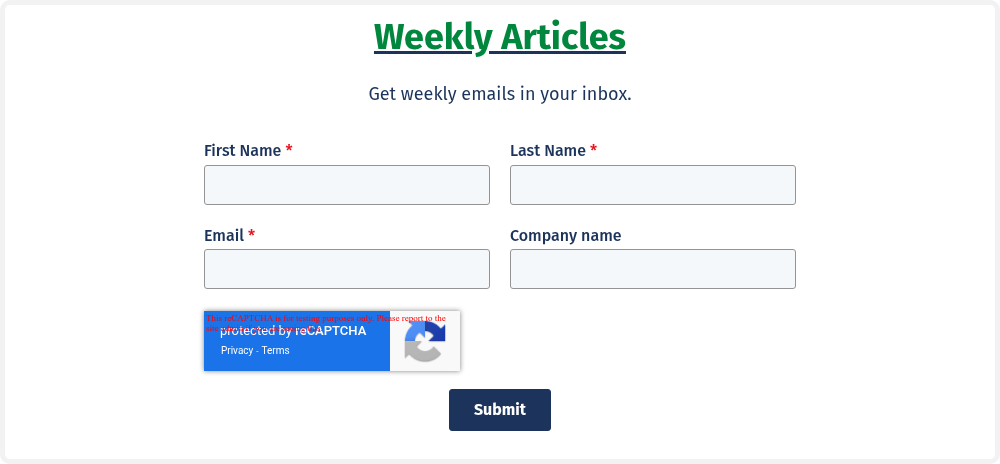