TL;DR • The terminology – “audit” vs “review” - is important, but clarity about deliverables is...
Algorithm Reviews: Public vs Private Reports
If you've encountered the view that "AI audit reports" need to be made public, and you're responsible for an algorithmic system, you might feel a bit uneasy.
However, for most readers of this article, there's no need to be overly concerned.
This article explains why you shouldn't be too worried, and how to redirect the energy misspent on anxiety.
Context Matters
AI and algorithm audit guidelines vary widely and are not universally applicable. We discussed this in a previous article, outlining how the appropriateness of audit guidance depends on your circumstances.
Here are some further specifics to explain what this notion of public audit reporting may mean for your situation.
Where this comes from
Public financial statement audit reports are often cited in the context of AI audits. Here is some background information on financial audits that will help in understanding the potential direction that AI audits will take:
- Historical Context: public financial audit reporting emerged in the early 20th century, driven by the need for transparency and investor protection.
- Applicability: public reporting typically applies to publicly traded companies and certain large private entities.
- Exemptions: small businesses and most private companies are often exempt from public reporting.
- Time lapse: the current financial audit regime developed over decades, responding to changing governance requirements and corporate scandals.
AI audit reporting may follow a similar path.
It will probably happen faster. But it is reasonable to assume that it won’t happen overnight.
Emerging Legislation
While public reporting isn't universally required, recent legislation shows some signs of moves in that direction:
- EU AI Act: mandatory audit expectations, with potential public reporting.
- Colorado ECDIS Act: requires insurance companies to consider external data/algorithms, and file annual reports with the state.
These signal a growing trend towards transparency.
The EU AI Act primarily targets high-risk applications (in terms of audit requirements).
The ECDIS Act requires annual returns, but not necessarily public audit reports.
Audit vs Review
We explored this topic in depth in a previous article. Here are the key points relevant to our discussion:
- An audit is a specific, formal process typically conducted by an independent external party. It follows a defined methodology. The result is a report that may be used for compliance or certification purposes.
- An audit can be considered a type of review. But there are other types of reviews too. They produce documents or reports for internal use, identifying what’s working and what needs to be addressed.
This distinction is important. The debate around public reporting relates to formal audits, not other types of reviews.
Understanding this should alleviate some anxiety about public reporting. If you're conducting internal reviews of your AI systems, these are likely not the “audits” discussed in the context of public disclosure debates.
Transparency demands mainly target formal independent audits. And primarily for high-risk AI applications.
When Public Reports Might Be Necessary
The need for public reporting will be driven by several factors, including:
- Legislation: specific laws may require public disclosure of the results of certain algorithm audits.
- Transparency: Your organisation may choose to be transparent, as part of its ethical or public relations strategy.
However, this won't apply to all reviews.
And when public reporting is required, the level of detail in a public report is likely to differ from an internal review report. This is because:
- Some information needs to remain confidential
- Not all aspects of a review need to be reported on publicly
- Some findings may not be appropriate for public disclosure
- Many reviews are conducted to proactively address issues.
For example, if you commission a review of your algorithmic fraud system to check for potential bias, you might choose to publicly share some information. But you won’t disclose all the details. For example, how you check for fraud may need to remain hidden, or it may be easier for fraudsters to figure out how to bypass the checks.
Note: for high-risk AI systems that fall under the EU AI Act, certain information may need to be registered in the EU database. This includes a description of the system, its intended purpose, and information about the provider.
Preparing for Potential Public Reporting
Even if your organisation isn't currently required to publish AI audit reports, it's wise to be prepared.
Here are three things you can consider now:
- Ethical AI Practices: You're likely already implementing or contemplating robust AI governance practices. If not, now is a good time to start. This type of commitment will position you well for any future reporting requirements.
- Regular Reviews: Conduct periodic assessments for fairness, accuracy, security, privacy and other risk areas. These help you to identify issues to resolve, early. Even if the issues are not complicated, they can take time to fix.
- Reporting Strategy: Consider the information you would be comfortable sharing publicly if required. A proactive approach can help you prepare for potential future reporting requirements, with safeguards, for example to maintain confidentiality.
Where to from here
The potential for public AI audit reports shouldn't be a source of anxiety. Instead, view it as an opportunity to strengthen your AI governance practices.
While there's a growing trend towards transparency in algorithmic decision-making, especially in the public sector, the need for public reports on algorithm integrity reviews is not universal.
However, banks and insurance companies using high-risk AI systems, as defined by the EU AI Act, may be subject to specific requirements. Further public reporting requirements may emerge as regulators gain more experience in overseeing these systems.
So, being prepared is important. This includes identifying and mitigating risks before they become issues.
By conducting regular, thorough reviews of your algorithmic systems - you can ensure their integrity, fairness, and reliability.
Disclaimer: The information in this article does not constitute legal advice. It may not be relevant to your circumstances. It was written for specific algorithmic contexts within banks and insurance companies, may not apply to other contexts, and may not be relevant to other types of organisations.
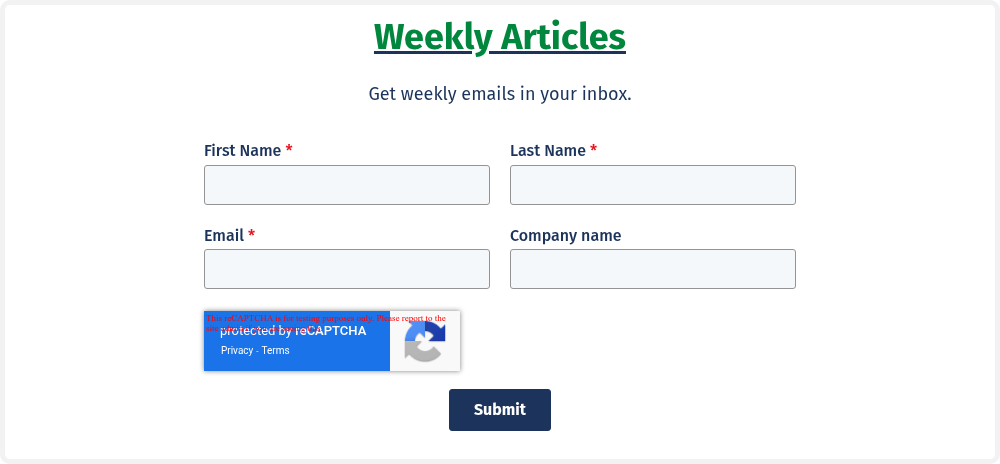