In-Flight vs. Post-Implementation reviews: model build and refinement Data-driven (or data-enabled)...
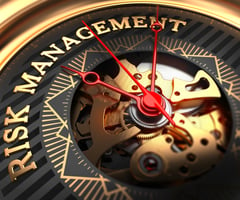
They can be manual, reliant on reports, semi-automated or fully automated.
They typically look something like these:
Reports with data from multiple sources, that you rely on to make operational decisions (e.g., sales KPIs)
Regular reports that you use in operating key controls (e.g., reconciliation reports)
Regulatory reports (e.g., capital adequacy calculations)
3rd party commission calcs that you use for external payments (e.g., to brokers for loan origination)
Fraud risk rules and models, flagging potentially fraudulent interactions (e.g., fraudulent insurance claims)
Automated fee or payment calculations without human intervention (e.g., automated bank fee postings).
There are many components that need to be designed (and programmed) perfectly.
One errant line of code or data quality issue can spell disaster.
How can you be sure that the inputs are correct?
Can you trust that the transformations are error-free?
How can you confirm that the flows align with their objectives?
Do your rules and models promote bias, or are they fair and equitable?
How can you be confident that your systems are generating accurate results?
We review those flows, at various levels of depth (depending on risk and your required level of confidence).
Our approach is based on first principles, starting with your expectations and testing from there.
This differs from the typical approach that focuses purely on the technical details.
We find that many errors stem from translation issues (requirements ≠ functional specs ≠ tech specs ≠ build).
Schedule an introductory call to discuss how we can help you achieve trust and confidence.
Ensuring the accuracy and integrity of your reports, providing comfort that you can rely on the content.
In-depth reviews of key process flows, incl. data sources, data transformations, calculations, models, etc.
Identifying potential biases in decision algorithms and processes, to promote fairness and objectivity.
In-Flight vs. Post-Implementation reviews: model build and refinement Data-driven (or data-enabled)...
Does testing General IT controls provide assurance over reports? This is a commonly held belief. It...
Many operational decisions are now heavily reliant on automation - data, models, rules etc. We’re...