TL;DR • Banks and insurers sometimes focus on business concerns and regulatory matters in assessing...
External data – use with care
Banks and insurers rely on external data in serving customers and making decisions.
Sometimes these are used deliberately, with care, in line with the purpose for which they were collected.
Other times, they are thrown into the mix because they are on hand, and seem to “improve” model performance, but not in alignment with their stated purpose.
Listen to the audio (human) version of this article - Episode 14 of Algorithm Integrity Matters
Regulatory signals
We’ve explained previously why we shouldn’t wait for specific legislation.
There are existing regulations that cover bias, anti-discrimination, etc.
But specific laws and expectations about responsible use of external data are emerging.
Colorado's External Consumer Data and Information Sources (ECDIS) law and New York's proposed circular letter, highlight a growing focus on potential bias and discrimination that stems from inappropriate use of external data.
The details vary, but both expect active oversight by boards and senior management to ensure that external data is used responsibly.
Other regulators may follow suit.
An analogy from another industry
Let’s consider a well-known example from the pharmaceutical industry that might be helpful.
It is certainly not foolproof, but we can learn from it.
Pharma has strict regulations. For example, medication package inserts provide information about a drug's composition, intended use, potential side effects, and contraindications. These are important for both healthcare providers and patients, promoting safe usage and informed decision-making.
Applying this to external data
Imagine if external data came with similar "data inserts."
These could include detailed information about:
- Data composition: Where the data originates, how it was gathered, privacy practices.
- Intended Use: Specific purposes for which the data is designed to be used.
- Potential Biases: Acknowledgment of any known biases in the dataset (similar to side effects).
- Validation Methods: A description of how the data has been tested for accuracy and fairness.
Intended use
As an example, some external data is intended to be used for marketing purposes.
They can, for example, help target segments of the population that our products and services will suit. There are considerations here, of course, like making sure they align with design and distribution obligations. But marketing is the purpose outlined when we get the data.
In medical terms, this might be like a prescription.
But let’s say we use the data for a different purpose – insurance pricing, for example.
Some marketing focused external data categorises people into demographic segments, so using it for pricing can result in discrimination (direct or proxy).
This could then be like prescription drug misuse.
Using medication for a purpose other than for which it was prescribed can be seriously risky.
The same can hold true for using external data for a different purpose.
Protecting against misuse
We don’t (yet) have consistent expectations for external data.
The new Colorado law and New York guideline will help, for those jurisdictions.
For everyone else, existing legislation still applies. Even if they’re not that specific.
We must protect our customers, using the data safely and responsibly.
To achieve that, here are some questions that we can ask. Some appear repetitive – this is deliberate.
Ask ourselves
- Are we aware of all the external data we are using, and where?
- What will our customers say if they know what data we are using, or what we are using it for?
- Have we clearly disclosed to customers that we use external data, and where we use it?
- If a customer wants to contest a decision that used external data, are we adequately prepared?
- How can we educate our teams to prevent misuse of external data?
Ask data scientists and developers
- How are we using external data?
- Are we using the data beyond its intended purpose?
- Have we used data just because "we have it”?
- Have we used data simply because we noticed a correlation?
- Do we have approval for each flow/model/algorithm we have used it in?
Ask data providers
- What is the purpose of the data?
- What should the data be used for?
- What should the data not be used for?
- Has the data been tested for accuracy and fairness/bias?
- How has the data been collected, and does that maintain privacy obligations?
Responsible use of external data
We’ve used external data for some time and will continue to do so.
We need to approach it with care, keeping our customers protected and complying with our obligations.
It starts with asking the right questions and always keeping our customers' best interests in mind.
Disclaimer: The information in this article does not constitute legal advice. It may not be relevant to your circumstances. It may not be appropriate for high-risk use cases (e.g., as outlined in The Artificial Intelligence Act - Regulation (EU) 2024/1689, a.k.a. the EU AI Act). It was written for consideration in certain algorithmic contexts within banks and insurance companies, may not apply to other contexts, and may not be relevant to other types of organisations.
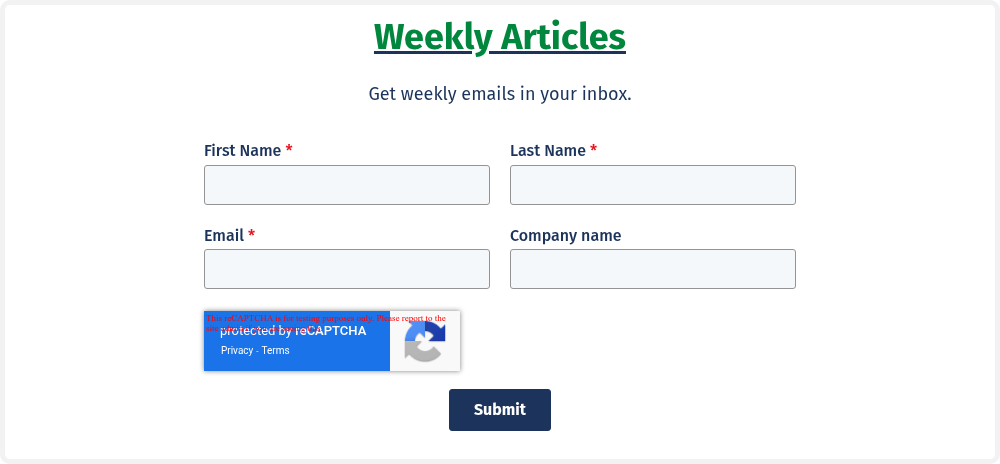